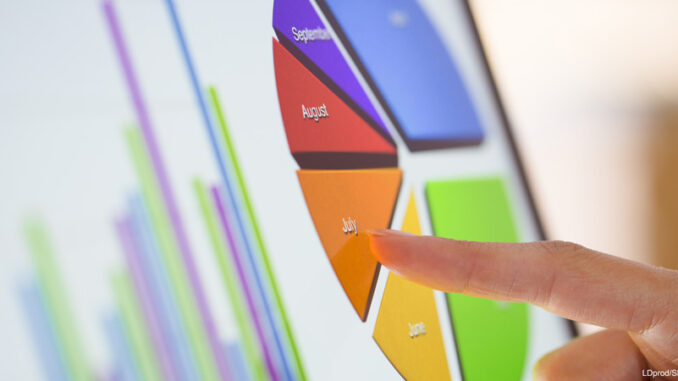
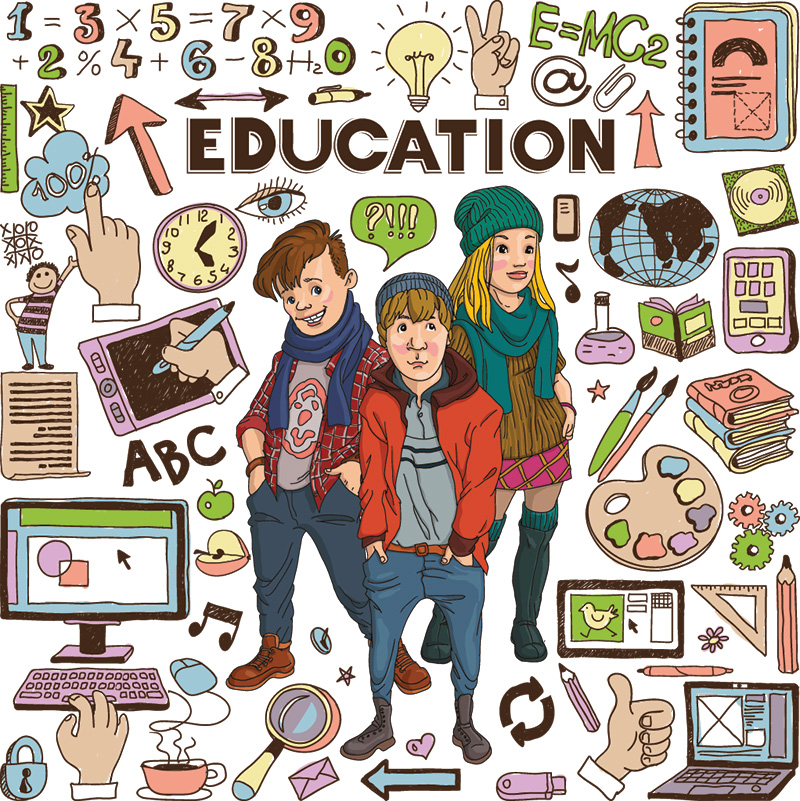
Have you started thinking yet about your plans for after you finish high school? For many students, a university is the next step. However, fewer than half of the nation’s students graduate college in four years, and only sixty percent manage to complete that education in six years. At the same time, the average debt accumulated by graduating college students last year was more than $28,000. Because of this, parents and politicians have started holding universities accountable and demanding better results. Universities are responding by using big data and predictive modeling to bridge the gap.
How It Works
Colleges and universities hire predictive analytics companies to examine thousands and even millions of student academic and personal records, both past and present, and identify trends in the data. They then create computer programs that will identify when a student is at risk and alert that student’s academic adviser accordingly. For example, an adviser might get an alert when a student receives a poor grade in a course needed for his or her major, or if a student doesn’t take a required course when he or she is supposed to, or if a student signs up for the wrong class. The adviser then sets up a meeting with the student to get him or her back on the right track.
In the past, advisers looked mostly at a student’s grade point average to determine his or her academic standing. But what predictive modeling has discovered is that some classes, such as a required course in a student’s major, are more important for predicting success or failure than other classes. For example, if a student majoring in political sciences receives two A’s in non-major classes, and a C in Political Science 101, his or her grade point average is still reasonably strong. However, that student will be much more likely to drop out or change majors later on than a political science major who received an A in the same introductory class.
But this type of data-gathering can go beyond the classroom. Predictive models can also be built to include analysis of students’ social interaction by tracking how often they swipe their ID cards to go to the gym or purchase a meal at the union. Students who are the most comfortable and socially integrated are the most likely to succeed, while those who are not socially integrated tend to drop out after their first year.
What Are the Drawbacks?
Most of the concerns surrounding predictive analytics have to do with the issue of invasion of privacy. (Think about it . . . how comfortable are you knowing that a statistician knows how often you might choose to buy snacks between classes?) However, the models work much the same way that Amazon or Facebook do. These extremely popular Web sites already examine your mouse click history and use it to predict a purchase you might wish to make or a page you might want to “like.” Others worry that a student who is “flagged” by the system will be labeled as at-risk, or will be discouraged from continuing on with his or her major if he or she receives a poor grade in an introductory course.
That’s why, for the universities who have implemented this program successfully, the data is only part of the equation. Schools–especially large public schools–have significantly increased (or, in some cases, doubled) their number of academic advisers. Other schools have begun free tutoring programs, or have used the data to redesign core courses. Though computer models can provide universities with indicators of a student’s future performance, ultimately the school must devote resources to the problem if they would like to see improvement.